Nvidia Kepler List
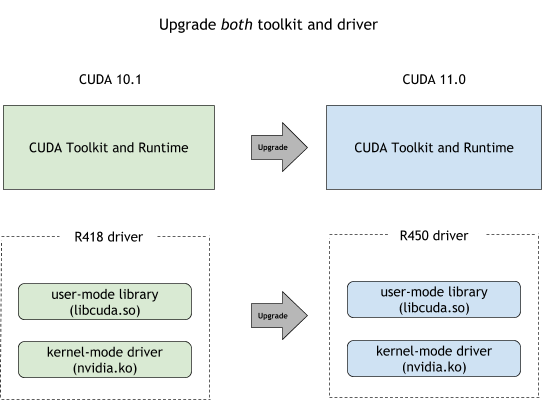
1024 mb gddr5 128 bit.
Nvidia kepler list. If you re running a recent version nouveau you can find your chipset by doing dmesg grep i chipset. In the meantime you can find a complete list of mobile kepler products over at nvidia s site. Product name gpu chip released bus memory gpu clock memory clock shaders tmus rops. Geforce 600 geforce gtx 645 oem.
It is suffice to say that only gpus with kepler architecture or newer are capable of accomplishing the deep learning tasks or in other words deep learning ready. 4 the geforce gt 705 oem is a rebranded geforce gt 610 which itself is a rebranded geforce gt 520. All but nvidia g80 have mostly been validated by checking more than one website if you see any mistake let me know. Most geforce 600 series most geforce 700 series and some geforce 800m series gpus were based on kepler all manufactured in 28 nm.
This list details all of the notebook parts based on the kepler architecture. 3 kepler supports some optional 11 1 features on feature level 11 0 through the direct3d 11 1 api however nvidia did not enable four non gaming features to qualify kepler for level 11 1. Kepler has powered a slew of mobile gpus as they weren t limited to the 600m series. 384 32 16.
Kepler was nvidia s first microarchitecture to focus on energy efficiency. Be aware that this list may be outdated and incomplete. This post summarizes the list of nvidia desktop gpu models that serve as a better fit for building a deep learning ai system. 1024 mb gddr5 128 bit.
576 48 16. There are some geforce gpus with different architectures while having the same name. This will always be correct whereas the lists below are approximate. Geforce gtx 560 geforce gt 630 geforce gt 640.
Kepler is the codename for a gpu microarchitecture developed by nvidia first introduced at retail in april 2012 as the successor to the fermi microarchitecture. The list is rather extensive along with the 600m series kepler parts were also used as part of. Data scientists and researchers can now parse petabytes of data orders of magnitude faster than they could using traditional cpus in applications ranging from energy exploration to deep learning nvidia s accelerators also deliver the horsepower needed to run bigger simulations faster.